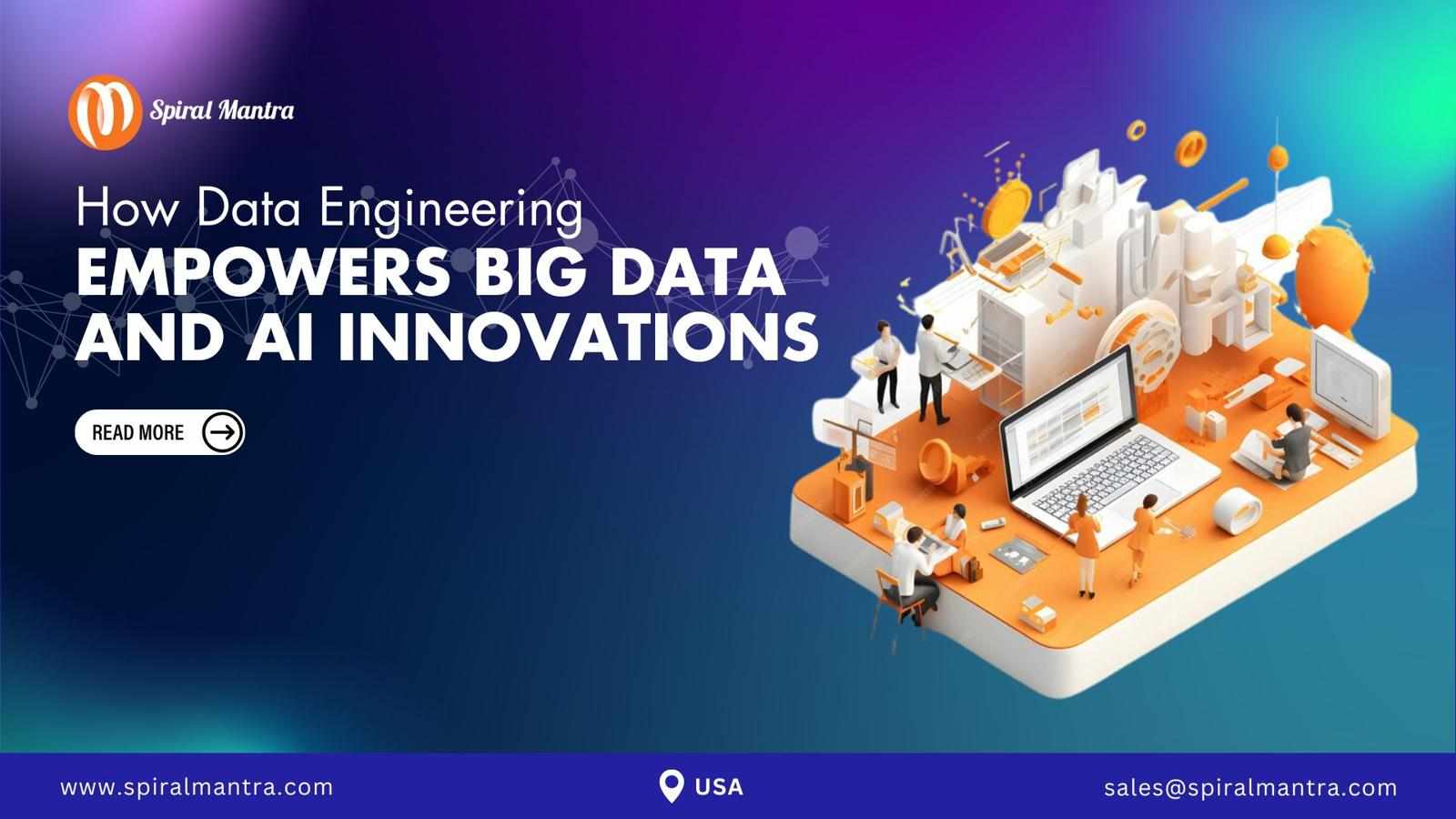
With the increasing adoption of Artificial Intelligence (AI), data engineering has transformed the way businesses manage and utilize data. As data has become a vital asset for organizations, there is a growing need for effective solutions that can help collect, modify, and analyze large volumes of information to derive impactful results. The combination of data engineering and AI has revolutionized the industry, providing businesses with the tools to optimize their operations and make data-driven decisions that shape their future strategies. This article discusses the pivotal role of data engineering in AI innovations and how it benefits organizations.
The Importance of Data Engineering in AI Adoption
Data engineering is at the core of the AI-driven revolution. It provides the essential framework for managing, processing, and transforming raw data into structured and usable formats. However, implementing AI solutions in data-driven environments comes with its set of challenges. These obstacles must be addressed effectively to leverage the full potential of both AI and big data analytics. AI can provide valuable insights, but it requires high-quality input data, a challenge many companies in the USA face due to poor data management practices. In this context, data engineering helps by ensuring that data is accurate, clean, and integrated, providing AI models with the right inputs to generate meaningful outcomes.
Roadblocks to Data Engineering and AI Adoption
Several challenges can impede the seamless integration of AI and data engineering. These challenges need to be addressed for businesses to achieve the maximum benefit of these technologies:
Access to High-Quality Data
One of the major hurdles companies face is the lack of access to quality data. AI models rely on accurate and well-structured data to generate reliable results. Without a proper data management strategy, businesses often struggle with poor data quality, making it difficult to train AI models effectively.Data Silos
Data silos refer to the practice of isolating data within different departments or systems, making it difficult to share and access information. This hampers collaboration and prevents businesses from gaining a holistic view of their data, which is crucial for effective AI deployment.Security and Privacy Concerns
With the vast amount of data AI requires, businesses must ensure the security and privacy of their information. Poor data security practices can lead to cyberattacks and data breaches, which can severely impact a company’s reputation and bottom line. Implementing proper security measures such as encryption and access control is vital to protect sensitive data.Complexity in Data Integration
The rapid accumulation of data can lead to inefficiencies in existing systems. Businesses often face challenges in processing large sets of data, which can slow down decision-making processes. Efficient data engineering helps overcome these challenges by creating streamlined data pipelines that allow for real-time processing.
The Benefits of Combining Big Data and AI
The fusion of AI and big data technologies has provided businesses with numerous advantages:
Automation of Repetitive Tasks
By combining machine learning and AI, companies can automate repetitive tasks such as data cleaning, organization, and integration. This reduces manual effort, saves time, and allows engineers to focus on more strategic and creative aspects of their work.Faster Data Processing
Big data technologies enable businesses to organize information into structured formats, resulting in faster data processing. Machine learning models can then run on this data to generate real-time predictions, enhancing operational efficiency.Predictive Analytics
AI-driven predictive analytics has gained widespread popularity, particularly with the rise of generative AI models. Predictive analytics helps engineers identify patterns in data and use those insights to forecast future outcomes, improving decision-making processes.Improved Data Management
AI and big data technologies help organizations efficiently manage vast amounts of unstructured data. Tools like Apache Spark, Google BigQuery, and AWS Redshift make it easier to store, process, and scale data pipelines, enabling businesses to handle enormous volumes of information with ease.
How Big Data and AI are Transforming Data Engineering
The role of big data in addressing traditional input processing challenges is significant. Previously, businesses struggled with analyzing and storing large volumes of data, but now, technologies like Apache Kafka and Flink allow engineers to process data in real-time, enabling more accurate predictions and outcomes. These advancements have made it easier to execute predictive maintenance, fraud detection, and other complex tasks.
Furthermore, tools like Apache Cassandra and MongoDB provide scalable storage solutions for unstructured data, allowing businesses to manage their data more effectively. This makes it possible for organizations to leverage the power of big data and AI to improve decision-making and operational efficiency.
Conclusion
In conclusion, the integration of data engineering and AI has revolutionized how businesses handle data. By utilizing modern data management techniques and AI-driven solutions, companies can unlock the full potential of their data, automate processes, and make informed decisions. If you’re looking for expert big data developers who can help your business harness the power of AI and big data, consider reaching out to specialists like Spiral Mantra. With their industry expertise, you can take your organization’s data capabilities to the next level and achieve better outcomes.
Write a comment ...